Artificial Intelligence is the New Center of the Universe
By: Aaron Cruz
In 2017, researchers from Google released a paper called Attention Is All You Need, describing a new kind of structure for Neural Networks that was found to be effective with language-related tasks. The following year, researchers from OpenAI published Improving language understanding with unsupervised learning, which detailed how these models could be trained to classify and, more importantly, generate text. This led to the creation of Open AI’s first Generative Pre-trained Transformer, or GPT. GPT generates text the same way your phone does when you continuously press the word suggestions above the keyboard. However, using machine learning, GPT was able to work with real English grammatical structure, maintain topics, and even produce real facts, like state capitals. However, it barely came close to producing a convincing string of text at a large scale. OpenAI would go on to improve their models by adding more neurons, filtering and improving their data, and optimizing their training processes. In June of 2020, OpenAI published “Language Models are Few-Shot Learners,” detailing their latest state-of-the-art model, GPT-3. GPT-3 could write full-scale news articles based on made-up headlines. It was also the first model OpenAI refused to release to the public, citing safety concerns. OpenAI went on to create GPT-3.5, a model improved with further training.
In March of 2022, OpenAI refined a new technique called Reinforcement Learning with Human Feedback (RLHF). With this technique, they were able to align models to specific attitudes, and orient them to specific tasks. In their paper Training language models to follow instructions with human feedback, they covered how this could be used to align GPT to instruction-based prompts. This way, questions and commands would be more likely to be followed with their actual (correct) answers. They called this new model InstructGPT. After that, they oriented a model towards human conversation, and, continuing their naming scheme, called it ChatGPT. For research purposes, they released a chat interface, where they let people interact with the model. OpenAI had created various public research previews before, but this was the most advanced so far, and the first one that was easy to interact with for the average person. In many ways, it is to Artificial Intelligence as Napster was to the Internet–the first practical tool available to the public that just so happens to use this new revolutionary technology. Not only did Napster, the first online music downloading service, disrupt several industries and change the way we experience media forever, it also showed people around the world that the Internet had an unbelievably large number of practical use-cases. ChatGPT became the fastest growing website of all time. Just like Napster, ChatGPT proved that AI could be intuitive, and unbelievably useful, bringing on the largest revolution in AI research ever.
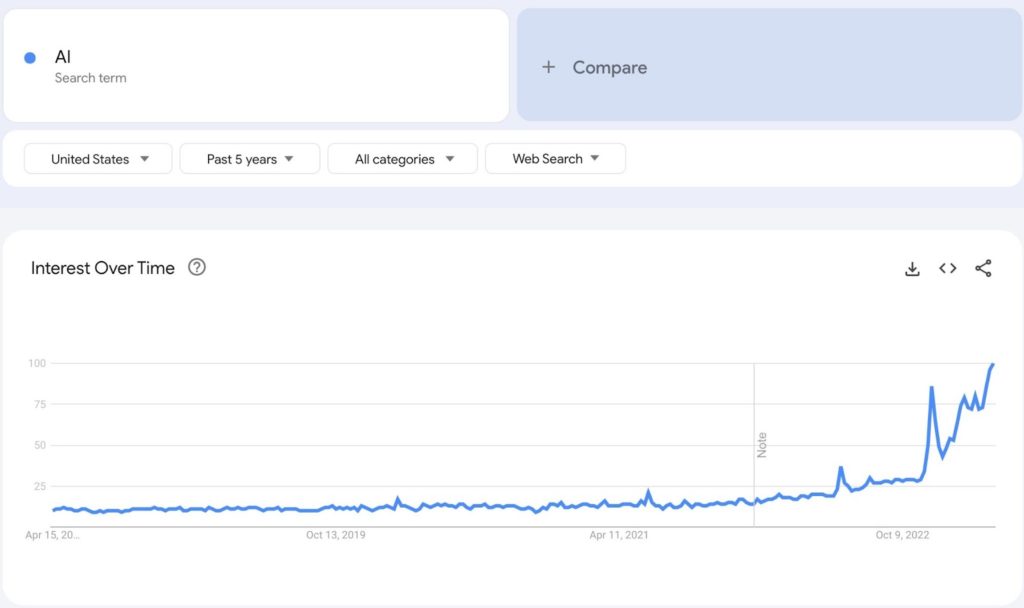
Interest in AI over the past 5 years.
But the most interesting part of AI isn’t the startups using it for new kinds of applications, or the companies looking to improve old ones, but the reactions people are having. In the short-sighted article Why AI can’t replace writers, published on Nov 9, 2022, blogger William Egan goes into detail about all of the things that AI writing tool Jasper failed to do when asked to write a copy for his job. He describes Jasper as “foolish” and “dumb”, and claims that it would be impossible for an AI-based writing tool to come up with novel ideas, and write a copy that could stand out from competitors. I chose this article in particular because it was released one week before the public release of ChatGPT. Egan’s next article, published two months later, is titled “How I Learned to Stop Worrying and Love ChatGPT.” In it, he claims that ChatGPT will replace any writing that isn’t “well above average.” In his next article: he finds a limit: ChatGPT doesn’t know facts. He correctly points out that LLMs, like ChatGPT, have a faulty understanding of the world, and they can misremember information, just as humans can misremember facts. (See also: ChatGPT is a blurry jpeg of the web). Of course, always on an unlucky streak, Egan’s article would go on to be rendered incorrect within a week, this time by Microsoft’s ‘New Bing’, an AI program that can search for and ingest data from the internet.
On November 29, 2022, website stacker.com published an article called 15 things AI can (and can’t) do. In it, they say the following: “AI cannot answer questions requiring inference, a nuanced understanding of language, or a broad understanding of multiple topics. In other words, while scientists have managed to ‘teach’ AI to pass standardized eighth-grade and even high-school science tests, it has yet to pass a college entrance exam.” Indeed, artificial intelligence is trained to regurgitate information it has seen; critical thinking skills are far outside the range of what a simple attention-based transformer model can do. It wasn’t just journalists though – Bill Gates claimed that an AI wouldn’t be able to do the amount of critical thinking required to pass an AI Biology exam for at least two or three years. (In hindsight, the inability of these humans to see what was going to happen next makes the pattern-recognizing capabilities of computers pretty impressive.) On March 14th, 2023, OpenAI published another paper, simply titled “GPT-4 Technical Report,” detailing their latest state-of-the-art model, GPT-4. GPT-4 scored a 1410 on the SAT and a 5 on the AP Biology Exam.
We’re playing whack-a-mole. We point to the capability, the idea, the magic sauce that makes us human, until someone figures out how to model it with a computer. If it isn’t combining complex ideas, connecting with other people, or coming up with new ideas and ways to solve problems, then what is it? What is the sauce? When we first gained consciousness as a species, we believed that the world was designed for us. The stars in the sky were there for us, and we lived in the center of the solar system, because we are divine. Well, as it turns out, if you take a closer look at how the stars behave, it seems more like they’re just, kind of, there. We are just another planet in our solar system. We evolved this way because we’re pretty good at reproducing and protecting our offspring. Bananas are easy to hold because things that get held get transported, moving their seeds around. As technology evolves, we see more and more that we aren’t special. For the first time, as computer science and psychology collide, we may be beginning to see the mundane-ness of our own minds. Human brains aren’t special, that’s just what happens when you put electricity in that formation. To what extent are you a transformer model, just guessing what word you want to say next? Really think of an answer. Think of it in English words, something you write down. Got it? Yes, AI language models are unfeeling things, just playing autocomplete, but, picture the second to last word in your response. Did you really think about what word to pick when you did? Do you really think about any words, at all?
The idea that your skill, something that you learned and honed for years, can be replaced by a computer, is scary. The idea that anything that anyone has done, all of the great poems, philosophies, and inventions, are mathematically simulatable, is existentially terrifying. When people make claims about the faults of artificial intelligence, I believe that many of them are doing so out of fear.
Online, everyone has an opinion on AI. What it does, how it works, the ways it can be trained, all of it. In non-technical fields threatened by machine learning, conversations always follow a similar format. People describe a tool, find issues with its output, and then position those issues as the final impasse that will keep their jobs safe. In marketing, it’s creativity, human relationship building, and intuition, all things that have already been matched, and in the case of relationship building, surpassed by GPT-4. In teaching, it’s learning from students, and utilizing new teaching styles, two things that most teachers can’t even do. All of these articles also ignore the extreme benefits of using an AI over a human being–computers never get tired, they never have anywhere else to go, and they work several orders of magnitude faster for a fraction of the cost. I won’t tell you whether hiring an AI marketer will be more effective than hiring a human, but for a moment, consider you are actually making the decision, with cost as a major motivator. Just at a glance, what is the better option?
When people can’t find a problem with AI output, they turn to finding reasons the technology shouldn’t exist in the first place. Yes, Midjourney can produce hyper realistic results better than any human, and, for anyone who needs an image for their project, it’s a better option than hiring someone. So the discussion around image generators turns to the way they actually create them–all the images the developers are training their networks on. Forget about human nature, that battle is over–stop the problem at the source and ban the technology. I won’t tell you whether or not I think the argument over the legality of AI-driven image generation is valid. But it’s interesting that now, now that a large number of human jobs are in danger, this is when we’re having these discussions. Nobody spoke up during the release of Google Translate, Grammarly, or img2img. Those were tools. Image generation? That’s theft.
No matter what people say, or how much people claim to understand generative models, there really is no way of knowing. At the beginning of the article, I talked about how OpenAI improved their models by “adding more neurons, filtering and improving their data, and optimizing their training processes.” Not one of those things involves working with the actual function or abilities of the models directly. No one’s been able to look at a model and say “that there–that’s the part with facts about Taylor Swift, and that’s the part that differentiates between there, their, and they’re.” Even discovering the neuron in GPT-2 that handles the difference between “a” and “an” is a monumental research task. The only thing we’ve learned about improving neural networks, after seventy years of AI research, is that the models get better when we make them bigger. GPT-2 (1.5 billion parameters) can regurgitate information, GPT-3 (175 billion parameters) can convincingly talk like a human, GPT-4 (1 trillion parameters) can (seemingly) do complex reasoning. That’s about the best we can do. We don’t know where the ceiling is. We never have. We might hit it at any moment. The limit might be your job! It also might not. There’s no way of knowing; and anyone who tells you they know, is lying.
Which is why it’s interesting to see the response from people who are “professionally smart.” Just look at the huge number of articles on “prompt engineering,” the practice of designing prompts for language models to get them to a specific task. All of these articles present the same ten facts, and the ones that don’t, make things up. We don’t know how the models work, so the best way to do any kind of prompt engineering is well-intentioned guess work, also known as the scientific method. For the first time, software engineers have encountered a piece of software that we don’t completely understand, and instead of acknowledging that, and adjusting our processes of communication, they have bypassed the need for rational discussion. The articles you’ll see online about how to better use AI tools are mythology, not science. The desire to conquer the technology is strong–just like cryptocurrency, there’s a notion that the people who make an effort to understand the technology now will become rich and powerful in the future. In the case of AI though, in a world where machines can outperform humans, the humans that have learned how to understand the machines will be replaced as well. Many of these new AI-hobbyists share the idea of the “irreplaceable replacer,” the idea that AI will replace the jobs they’re learning to automate, but they themselves could never be replaced. It’s just another layer of denial of the promise of AI.
When people like Jonathan Blow and Adam Conover make comments on artificial intelligence, they fail to, or intentionally omit, the striking amounts of progress that has been made in progress towards human-level A.I. As people witnessing the death of the myth of human genius, they are going through their own personal five stages of grief. Instead of accepting that you will probably be replaced, at the moment, the best we can do is accept that we don’t know if AI can or can not outdo us. (Blow has already reached that point.) The most thoughtful piece I’ve seen on the recent wave of AI is Tom Scott’s. At the end of his (fantastic) video, he says this:
“It’s not about ChatGPT, not specifically. It’s about what it represents. Because if we’re still at the start of the curve for AI, if we’re at the Napster point, then everything is about to change just as fast and just as strangely as it did in the early 2000s, perhaps beyond all recognition. and this time I’m on the wrong end of it. I’m like the music executive, back in ’99. It feels, to me, like something might have just gone very wrong for the now-comfortable world that I grew up with
and that I’ve settled down in. That’s where the dread came from. The worry that suddenly I don’t know what comes next. No-one does. I’ve been complaining for years that it feels like nothing has really changed since smartphones came along, and I think that maybe, maybe, I should have been careful what I wished for.”
For the past 15 years or so, we’ve known, essentially, the slate of upcoming technology. Now, this game changing tech, that invalidates a core belief of our species, is rocketing through progress at an unprecedented rate. The worst part is that we don’t know where that progress will end. No one does.
Your style is convincing, it’s difficult to look away.